This week focused on finishing up documentation, a little bit of cleanup and one final task of implementation that would be helpful.
DISTINCT
The SELECT DISTINCT clause currently uses a DEDUP(x,ALL)
, which is notoriously slow for large tasks. Instead an alternative clause was suggested
TABLE(x,{<cols>},<cols>,MERGE);
//eg. for a t1 table with c1 and c2
TABLE(t1,{t1},c1,c2,MERGE);
This looks good!
But, the question is – how to get the columns c1
and c2
for the table t1
? It may not always be known, or worse, the known type information may not be complete. Here, we are presented with two ways in which we can proceed with this information:
- Stick with DEDUP all the time. It is slow, but it will work.
- Use the new TABLE format, falling back to the DEDUP variant if type information given is not sufficient.
This is great, and it also incentives typing out ECL declarations, but it still feels as a compromise.
Template Language
Everything changed when I realised that ECL has a wonderful templating language. For a quick idea on what the point of a template language is, it can be used in a way similar to the preprocessor directives in C – macros that can be used to write ECL.
So, what can we do here? The good thing about writing macros is that since they are based off the same solution, the macro processor can work with data types in ECL very well, and also, can make ECL code.
So, can we write an expression that creates the c1,c2
expression, given that the table t1
is given?
__dedupParser(la):= functionmacro
#EXPORTXML(layoutelements,la);
#declare(a)
#set(a,'')
#for(layoutelements)
#for(field)
#append(a,',');
#append(a,%'{@label}'%);
#end
return %'a'%;
#end
endmacro
Although I won’t go into the details of this interesting function macro (A macro whose contents are scoped), in essence, it can take a record, and put out a snippet of code that contains the columns delimited by comma.
Using __dedupParser
Although the naming isn’t accurate, we can inspect what the macro does by an example
Given a record R1
, which contains two fields, f1
and f2
(Assume both as integer), then __dedupparser(r1)
will create an ECL code snippet of “f1,f2,
” (Notice the trailing comma). This works nicely with the RECORDOF
declaration, which can help get the record associated with a recordset definition.
So, this brings something really useful, as we can now have this general code syntax –
TABLE(<t1>,{ <t1> }#expand(__dedupParser(RECORDOF( <t1> ))),MERGE);
This simple expression generalizes the entire TABLE function pretty effectively.
Adding this into code, it is important to remember that the function macro needs to be inserted separately, and most importantly, only once in a ECL file. This is better done by tracking whether a DISTINCT clause has been used in the program (Using an action like we had done earlier), and inserting the functionmacro
definition at the top in this case. And with this, a good new improvement can be made to the SELECT’s DISTINCT clause.
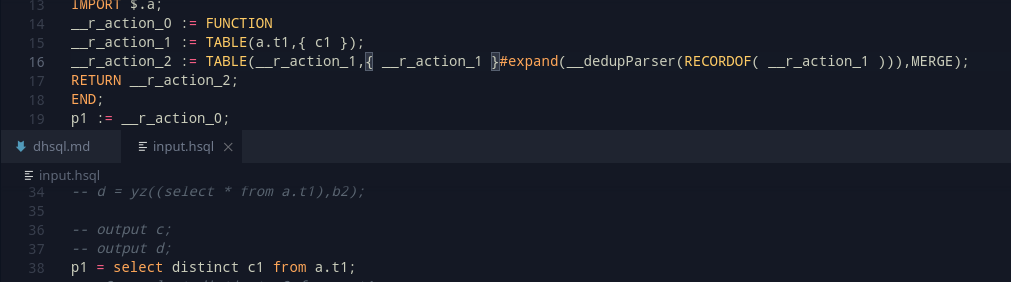
This should perform much better, and work more efficiently.
Documentation
So far, some of the syntax was stored and referred personally by the use of notes, memory and grammar. Writing this down in essential as a way of keeping a record (More for others, and even more importantly, for yourself). So, keeping a document to denote the syntax available to the user is rather important.
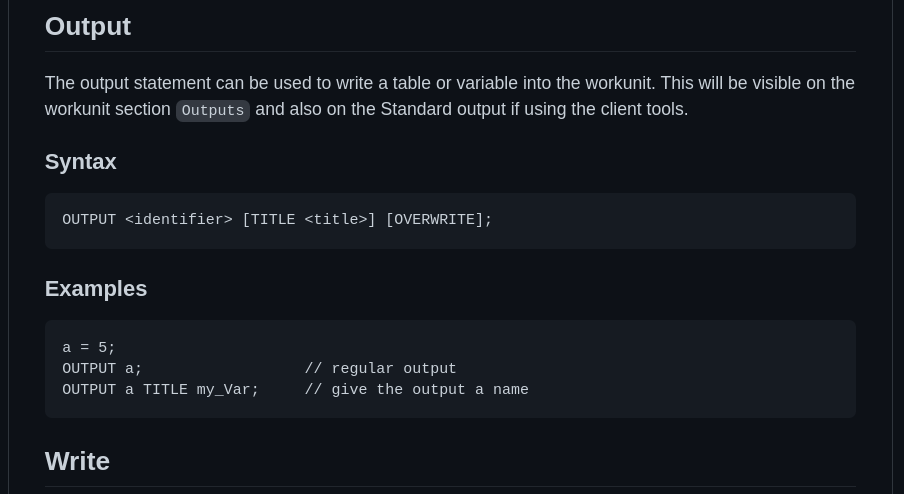
Here, its important to lay out the syntax, but also present some usable examples that an explain what is going on with that code segment. (Yeap, for every single statement!)
Winding up!
With documentation, that ends a lot of the work that was there. In fact, its already Week 12, although it is still surprising how quickly time has passed during this period. My code thankfully doesn’t need extensive submission procedures as I was working on the dev branch, and work will continue on this “foundation”. It has been a wonderful 12 weeks of a learning and working process for me, and I would like to thank everybody at the HPCC Systems team for making it such an enjoyable process! Although this is all for now, I can only hope that there’s more to come!